Recently, the Future of Life Institute published a letter asking for a six-month moratorium on “training AI systems more powerful than GPT-4,” with high-profile people including Elon Musk, Steve Wozniak, and Turing award winner Yoshua Bengio signing off. Though there are several sound recommendations, the focus shouldn’t be on fear and hypothetical risks, but rather on responsible regulation that enforces transparency of the technology.
AI is an incredible advancement in tech that’s new and still needs to be fully flushed out in terms of regulation, but the prominent viewpoints until now have largely been black or white.
Whether you’re for or against AI’s rapid development, it’s advancing and integrating into several industries, so it’s crucial to ensure that AI is developed, deployed, and used responsibly from the start. In fact, responsible AI is ensuring that AI algorithms do not promote bias or discrimination against any group of people. In fact, it is about driving diversity and promoting equitable outcomes.
Reducing bias in AI algorithms
This can arise from several sources including data sets, algorithms, and the people who design and train them. Responsible AI ensures that biases are identified and addressed so that the resulting algorithms do not unfairly discriminate against certain groups of people.
One way to achieve this is by using diverse, representative, and unbiased datasets to train AI systems. This means that the data should include a wide range of examples from different populations and contexts and be free from any discriminatory or prejudicial biases. If AI systems are based on unbiased datasets, that can give organizations an advantage from a legal perspective—the technology can help with compliance and inclusivity if deployed correctly and responsibly. If data inputs are biased then the output will also be biased, so it’s critical to identify those bottlenecks from the start. AI developers can use techniques like data augmentation, data balancing, and data cleaning to further reduce biases in the data.
Promoting and enforcing diversity
AI technology should be designed to ensure that all individuals, regardless of their background or identity, have equal opportunities and are included in search results. Pulling from a large data set alone doesn’t guarantee better diversity—algorithms should be trained to holistically evaluate candidates. For instance, assessing candidates on skills and experience rather than on degrees broadens the talent pool. AI technology should also indicate the reasons why a candidate was selected and why they are a good match.
In its annual report to investors, Micron demonstrated its commitment to increasing diversity in its workforce by using AI to mitigate potential biases from resumes. This ensures that individual merit and qualifications are prioritized over personal characteristics.
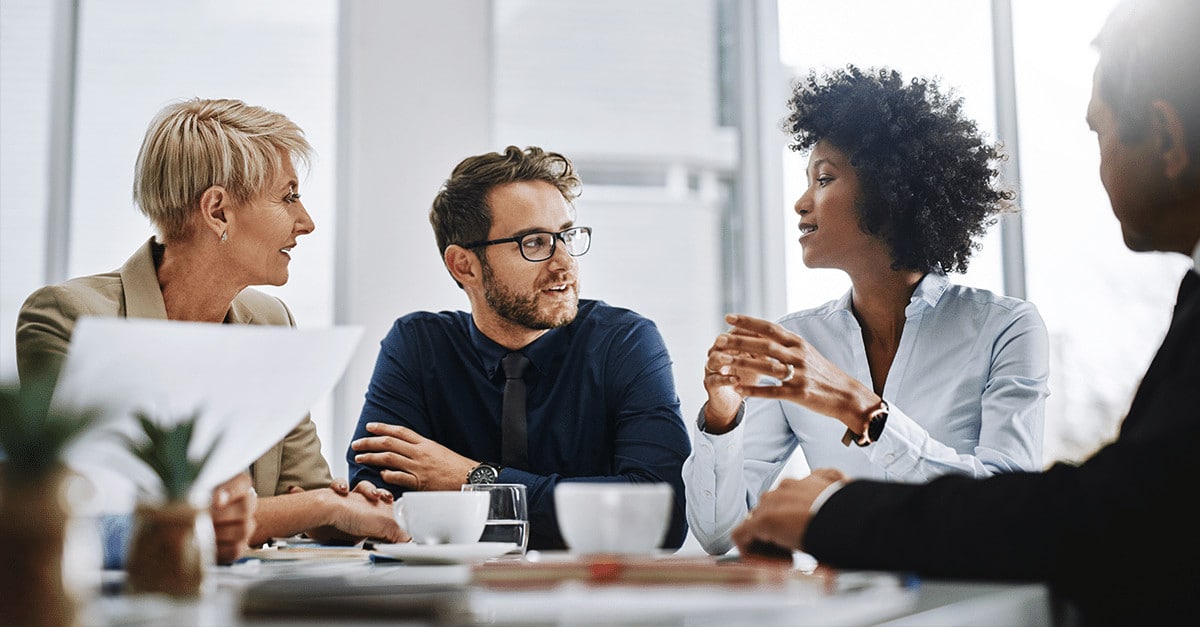
According to Keith Sonderling, the commissioner of the U.S. Equal Employment Opportunity Commission (EEOC), AI has not altered longstanding civil rights laws such as Title VII and the Americans with Disabilities Act (ADA). Instead, Sonderling suggests that these laws can provide valuable guidance to organizations seeking to comply with regulations while leveraging AI’s potential benefits.
Making HR processes more transparent and comprehensible
AI has the potential to be a valuable tool for organizations. However, it is incumbent upon these organizations to fully comprehend the technology so that they can correctly interpret its results. Only then can they take full advantage of AI and use existing laws as guardrails when integrating it into their operations. As Craig Leen, former director of the Office of Federal Contract Compliance Program (OFCCP) and leading expert on workforce compliance, equal opportunity, and anti-discrimination explains, “the less you can explain a particular AI’s outcome, the more valid that concern. The opposite is also true though, particularly for good AI.”
It’s critical for organizations to consider how they will use AI to compete in today’s marketplace and how it can enhance processes already in place, but the key is for potential buyers to truly understand the AI technology behind the buzzwords. Not only should it always be clear when a system delivers unverified or synthetic information, but organizations should request specific documentation on the underlying biases of training data and model architectures.
Understanding AI will help to identify potential areas of bias and ensure that the technology is used to promote diversity and inclusion. This will help to create a more equitable workplace where all people can thrive. Organizations that embrace responsible AI are more likely to have diverse and inclusive workplaces, which can lead to increased innovation, creativity, and productivity. Responsible AI is not a legal requirement, but it is essential for driving diversity and promoting equitable outcomes. Reducing bias is not new, but in the context of AI, it is more important than ever. Rather than getting wrapped up in the dangers of the technology, it is important for people to understand how AI really works—and that includes the risks, limitations, and potential outcomes.
Sania Khan is the chief economist at Eightfold AI, the AI-powered platform for all talent, and the author of the upcoming book Think Like an Economist. She previously worked for the U.S. Bureau of Labor Statistics.
This article originally appeared on Fast Company in April.